We turn biological insights into novel drugs
We use artificial intelligence (AI) and machine learning (ML) to interrogate our data in an unbiased, hypothesis-free manner to drive drug development
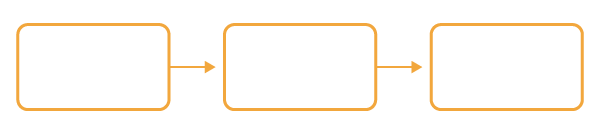
We let the data speak
We tarG3T data
We do not make assumptions or have a priori hypotheses. We use a combination of different analytical tools in a stepwise fashion to unravel novel biology, including:
Univariate analyses • Multivariable analyses: gradient boosting techniques • Knowledge-led pathway analysis • Bayesian network analysis • Genome-wide association studies • Mendelian/natural randomization studies
We identify panomic disease networks
We unravel entirely novel biological pathways using Bayesian network analysis
During Bayesian network analysis we allow clinical parameters such as age and gender to interact with a very large number of DNA variants, which in turn drive the panomic networks from above, including our panomic data of gene expression, proteomics, metabolomics and lipidomics. These molecular networks then drive the development of disease.
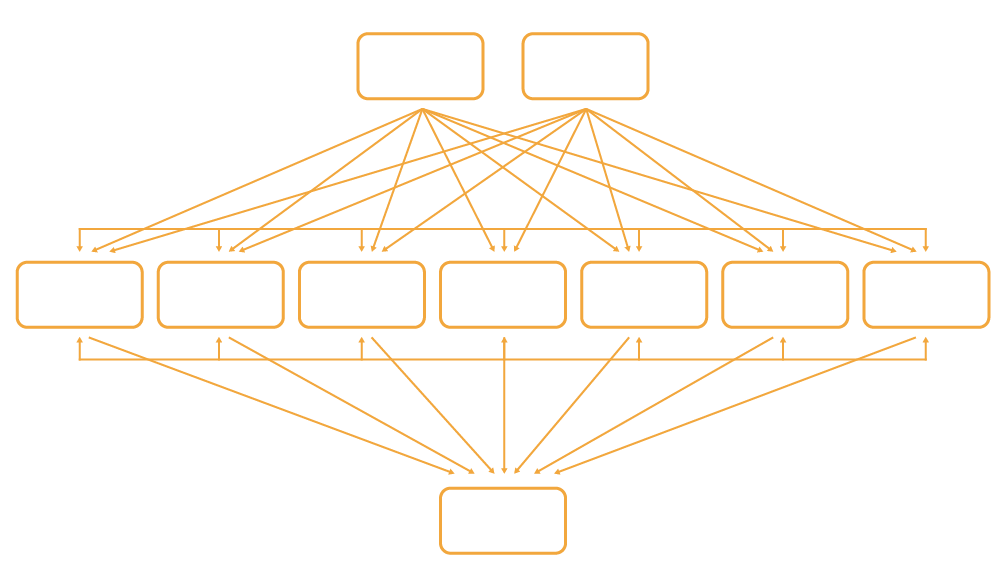
G3netic target validation
We double the chance of success by identifying genetic variants in causal genes associated with both a causal biomarker and the disease phenotype of interest
Once we have identified a potential drug target the last and very important step is genetic target validation (“G3TV”). Our genetic target validation approach is anchored in “natural randomization”, also called “Mendelian randomization”. The 3 key elements of Mendelian randomization align with the 3 pillars of the G3T platform and our genetic target validation approach:
• Precise, quantitative phenotype trait measurements for the relevant disease •
• A very large number of biomarker measurements •
• The whole genome sequence data •
There are 3 sequential steps required to establish causality
Step 1: Establish a statistically significant association between the disease phenotype in a patient population and the proposed causal biomarker.
Step 2: Identify genetic variants (SNP’s) that are significantly associated with high levels of the proposed causal biomarker (“GOF” variants) and low levels of the causal biomarker (“LOF” variants).
Step 3: Use genomic data to establish a significant association between the presence of SNP's and the disease phenotype within the population, thereby validating the causal SNPs.

Genetic target validation improves the probability of success by a factor of 2
G3T has successfully utilized this approach on several different occasions and consequently has a number of assets currently in preclinical development
Deep
learning
Take EVERY panomic and molecular datapoint as above and generate scientific insights in an unbiased, hypothesis-free approach.